AI at Scale: Training Models and Unlocking Value
Successful deployment of AI extends requires a strategic approach to data management, leveraging emerging DataOps practices and fostering collaboration across the data ecosystem.
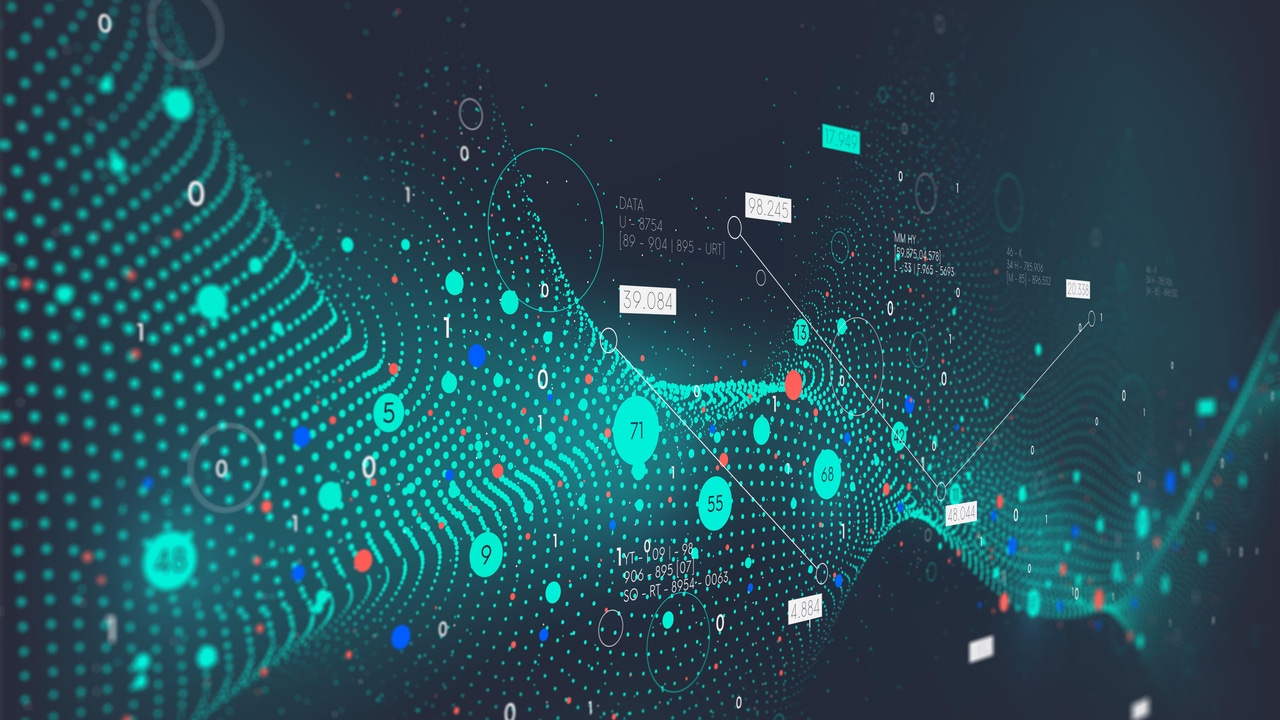
For most organizations, increasingly complex IT environments present a conundrum. While there’s more data available within the organization, it is often siloed and requires expertise from different technology domains to decipher. Not only is it challenging for human minds to effectively handle that complexity, but it is also not feasible to efficiently scale resources to analyze the volume of data. .
Yet, to stay competitive and manage complexity, organizations need to unlock the value of AI at scale. They can’t do it without data – data is the oxygen that AI needs to function. A 2024 survey from Wavestone found that 87% of executives reported getting value from their data and analytics investments. That's a marked increase from the 2017 version of that survey, when just 48% of executives held that opinion.
We are increasingly seeing differentiation among companies – between those that can deploy AI successfully and thrive and those that lag behind because they cannot. And while AI is seen as a business imperative, deployment is not as smooth as most would like. Just 37% of companies reported that efforts to improve data quality have been successful – an indication that while companies are seeing value in some projects, they may not see value in all of them (or have to spend excessively to get value).
So, how can organizations deploy AI at scale to unlock value?
First, they need to ensure that there is high-quality, highly-available data to train AI models. Next, organizations need to unleash this AI on vast data sets for the use cases under consideration to solve IT problems at scale, prove the value, and provide a basis for further iterations. Let’s dive deeper.
Ensuring High-Quality Data
There are several barriers to building a high-quality data pipeline that offers ubiquitous availability. Some are perennial and common to many organizations, such as inadequate collaboration between data producers and data consumers, or an unclear approach to measuring success.
There are also new challenges that have arisen in the age of AI. Traditional data management processes and practices don’t align well with newer technologies that AI enables, resulting in a process mismatch.
To ensure high-quality data, organizations need to automate and orchestrate data across heterogeneous pipelines, harmonizing data as it flows through multiple steps: ingestion, integration, quality testing, deployment, and monitoring, all while managing essential metadata, governance, and security.
Emerging DataOps practices, with their emphasis on applying the agility of DevOps workflows to data management practices, can help achieve these goals. With improved data pipelines, organizations will have a much easier time training AI models to meet their business needs.
Unleashing AI
Data and AI are inextricably linked. AI can be used to collate, contextualize, and analyze your organization’s data and then help you use it to learn about your business and your customers. With AI combing through data, you can uncover new insights that were previously inconceivable even a few years ago—and make informed decisions that drive competitive advantage.
Few organizations boil the proverbial ocean when it comes to the deployment of AI. Most start with pilot projects, where they can prove the value of AI quickly. From there it can be applied to broader use cases. What does this look like in action?
As an example, some organizations may use AI to monitor activities in real time in order to respond to IT performance and availability issues before they have a chance to impact the business. Prior to AI, this was time-consuming, laborious, and most insights were stale by the time the analysis was complete. These AI capabilities that quickly identify root causes for IT issues and even make recommendations on remediation enable organizations to free up IT teams to focus on more important tasks or drive innovation.
A best practice when operationalizing AI is to identify high-value projects and build a list of initiatives that will generate impact quickly. You can transfer that experience to more and more projects, mapping AI deployment to business impact. It is also critical to adopt a composite AI strategy leveraging a combination of Causal, Predictive, and Generative AI to maximize the potential of extracting insights and driving actions from data.
Forging a Path to Innovation
The journey toward harnessing the full potential of AI at scale is both promising and potentially fraught with challenges. As organizations navigate increasingly complex IT landscapes, the imperative to transform vast data reservoirs into actionable intelligence has never been more critical. Despite the hurdles of managing and deriving value from burgeoning data volumes, the trajectory is clear: organizations committed to optimizing data quality and embracing AI are distinguishing themselves, forging paths toward innovation and competitive advantage.
Successful deployment of AI extends beyond mere data availability; it requires a strategic approach to data management, leveraging emerging DataOps practices and fostering collaboration across the data ecosystem. As we venture further into this era, the integration of AI with data-in-motion promises to unlock unprecedented opportunities for real-time insights and strategic agility.
Related articles:
About the Author
You May Also Like